Hard Data vs Soft Data: Understanding the Differences
When it comes to data analysis, two terms often come up: Hard Data and Soft Data. These terms may sound technical, but they’re crucial in understanding how decisions are made in businesses, research, and even our daily lives. So, let’s dive into the world of hard and soft data and explore their importance.
- Hard Data vs Soft Data: Understanding the Differences
- Understanding Hard Data
- Understanding Soft Data
- Hard Data vs Soft Data: A Comparative Analysis
- The Role Data in Different Fields
- The Role of Data in Forecasting
- Hard Data, Soft Data, and Big Data: An Interconnection
- The Future of Hard Data and Soft Data
- The Interplay of Hard Data, Soft Data, and ROM
- In Conclusion
Understanding Hard Data
Hard data, often referred to as quantitative data, is the backbone of any data-driven decision-making process. It’s the type of data that can be objectively measured and quantified. This data is typically numerical and can be used for statistical analysis to draw precise conclusions.
For example, in a business context, hard data could include the number of units sold, revenue generated, or website traffic statistics. In a scientific research context, it could be the measurements taken during experiments, such as temperature readings or time durations. One of the main advantages of hard data is its objectivity. It’s based on measurable facts, which means it’s less likely to be influenced by personal feelings or biases. This makes it a reliable source of information for making informed decisions.
However, hard data also has its limitations. While it provides a clear and concise picture, it often lacks the depth and context that can explain why a particular trend or pattern is occurring. It tells us the ‘what,’ but not necessarily the ‘why.’
Understanding Soft Data
On the other side of the spectrum, we have soft data. This is qualitative data that is more subjective and interpretive. Soft data often comes from non-numerical sources and can include things like customer reviews, interview transcripts, or survey responses.
For instance, if you run a restaurant, soft data could be the feedback you receive from customers about the quality of your food or the ambiance of your establishment. In a research setting, it could be the personal experiences shared by participants during an interview. Soft data provides a richness and depth of understanding that hard data often cannot. It can help us understand the ‘why’ behind the numbers, providing context and insight into human behavior, emotions, and experiences.
However, the subjective nature of soft data can also be a disadvantage. It can be influenced by personal biases and is often more difficult to analyze and interpret than hard data. Furthermore, because it’s not typically represented numerically, it can be challenging to use soft data to make broad generalizations or predictions.
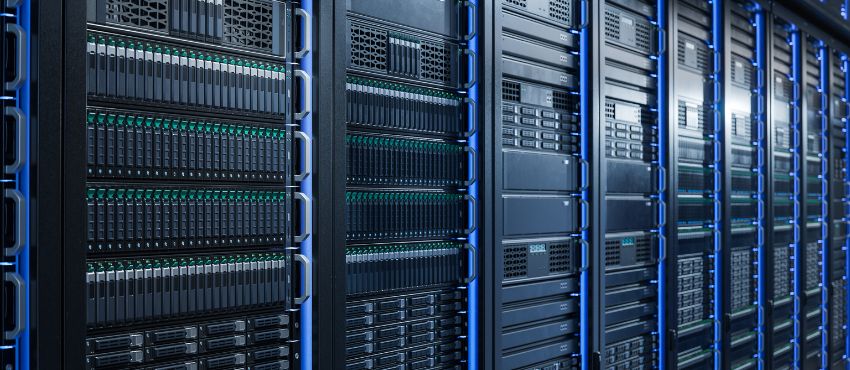
Hard Data vs Soft Data: A Comparative Analysis
When comparing hard data and soft data, it’s like comparing apples and oranges – they’re different, but both have their unique value. Hard data is objective, measurable, and numerical. It’s all about facts and figures. On the other hand, soft data is subjective and interpretive, focusing on human experiences and emotions.
Despite their differences, hard data and soft data often complement each other. For instance, in decision-making, hard data can provide the factual basis for a decision, while soft data can provide the context and human perspective. This interplay can lead to more balanced and comprehensive decisions.
The Role Data in Different Fields
Hard data and soft data find applications in various fields, each playing a unique role.
In research, hard data often forms the basis of empirical studies. It allows researchers to quantify phenomena and draw statistically significant conclusions. Soft data, on the other hand, is crucial in qualitative research, where the focus is on understanding human behavior and experiences.
In the field of economics, hard data such as economic indicators, financial statistics, and market trends are used to make forecasts and inform policy decisions. Soft data, such as consumer confidence surveys and interviews with business leaders, provides insights into attitudes and perceptions that can influence economic behavior.
In education, hard data can be test scores, graduation rates, and other measurable outcomes. This data can inform educational policies and practices. Soft data, such as student feedback, teacher observations, and case studies, can provide a deeper understanding of the learning process and help develop more effective teaching strategies.
The Role of Data in Forecasting
Forecasting, whether it’s predicting market trends, weather patterns, or customer behavior, often relies on both hard data and soft data.
Hard data, with its quantitative nature, forms the backbone of most forecasting models. It provides the raw numbers and trends that feed into statistical algorithms to predict future outcomes. For instance, a retailer might use past sales data (hard data) to forecast future sales.
However, soft data also plays a crucial role in forecasting. It provides the context and qualitative insights that can help refine forecasts. For example, customer feedback (soft data) might reveal emerging trends or changing preferences that could impact future sales.
Consider the case of a weather forecasting model. Hard data like temperature readings, wind speed, and humidity levels are fed into the model to predict future weather patterns. But soft data, such as observations of cloud formations or animal behavior, can also provide valuable insights that enhance the accuracy of the forecast.
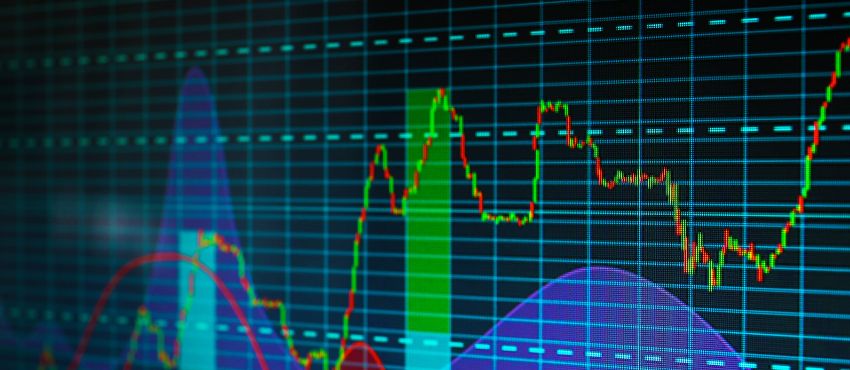
Hard Data, Soft Data, and Big Data: An Interconnection
In the era of big data, the lines between hard data and soft data are becoming increasingly blurred. Big data encompasses a vast array of information, from the hard data of numerical statistics to the soft data of social media posts or customer reviews.
The power of big data lies in its ability to combine hard and soft data to provide a more holistic view. For instance, a business might analyze social media sentiment (soft data) alongside sales figures (hard data) to gain a deeper understanding of customer behavior. Moreover, advances in technology are making it easier to quantify and analyze soft data. Sentiment analysis algorithms can turn subjective social media posts into quantifiable data. Similarly, machine learning algorithms can uncover patterns and trends in complex datasets, including hard and soft data.
The Future of Hard Data and Soft Data
As we look towards the future, the importance of hard and soft data is set to grow. With the increasing digitization of our world, the volume of hard data available for analysis is skyrocketing. Simultaneously, advances in technology are making it easier to collect, analyze, and interpret soft data.
Artificial Intelligence (AI) and machine learning are significant in this evolution. These technologies are not only helping to process and analyze large volumes of hard data more efficiently but are also enabling the quantification and analysis of soft data on an unprecedented scale.
For instance, natural language processing (NLP) algorithms can analyze text data from customer reviews or social media posts, turning this soft data into actionable insights. Similarly, machine learning algorithms can uncover patterns and trends in complex datasets, including hard and soft data.
In the future, we can expect to see a more integrated approach to using hard data and soft data. Businesses, researchers, and policymakers will increasingly recognize the value of combining quantitative facts with qualitative insights to make more informed and holistic decisions.
The Interplay of Hard Data, Soft Data, and ROM
ROM’s ability to handle both hard and soft data makes it a powerful tool for businesses. By combining the objectivity of hard data with the richness of soft data, ROM can provide a more holistic view of a business’s operations and customer behavior. This can lead to more balanced and effective decisions.
Moreover, ROM’s real-time features ensure that businesses have the most up-to-date data at their fingertips. This can be particularly useful in forecasting, where current and accurate data can significantly enhance the accuracy of predictions. Its comprehensive view of operations enables businesses to make more informed and effective decisions. Whether you’re dealing with hard data, soft data, or a combination of both, ROM has the tools to help you manage and analyze your data effectively.
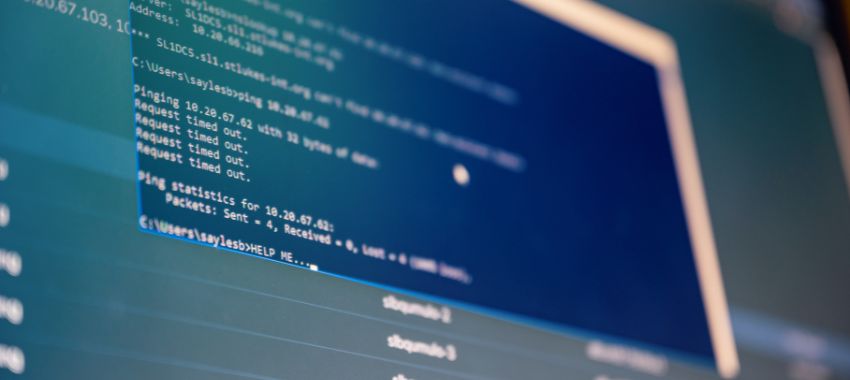
In Conclusion
Understanding the difference between hard data and soft data, and knowing when to use each, is crucial in today’s data-driven world. Hard data provides the factual basis for decision-making, while soft data provides the context and human perspective. Together, they offer a comprehensive view that can lead to more balanced and effective decisions.
As we move towards the future, the interplay between hard data and soft data, facilitated by advances in technology and AI, will continue to shape the landscape of data analysis and decision-making.